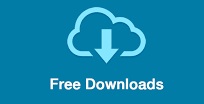
Select Start Media awarded the game 7.9/10, praising the unique idea of "tower offence" and solid gameplay. GamesBeat gave the game a score of 80/100, saying, "While it's hard to control, Anomaly is still playable and enjoyable as long as you take the time to really master the interface first.". Reception Īnomaly: Warzone Earth received strong reviews from critics.

A beta linux version was created but quickly scrapped as it was too buggy. The game was released on Xbox Live Arcade in April 2012.
#ANOMALY 2 VIDEO ANDROID#
An Android version followed, initially as an exclusive release on the Amazon Appstore, on November 29, 2011, then as a general Android Market release on January 25, 2012.
#ANOMALY 2 VIDEO PC#
In addition, the iOS and Android versions do not feature the levels set in Tokyo.Īnomaly: Warzone Earth was released on PC and Mac on Apand was released on iOS on Augas separate apps for iPhone and iPad. The iOS and Android versions omit the Commander in lieu of the player directly placing power using the touch-based interface. In the Windows, Mac, Linux and XBLA versions, players directly control a soldier on foot (dubbed the Commander) whom they use to pick up and drop the power-ups for the convoy. In addition, players can purchase and equip a number of different units with varying offensive and defensive attributes to make up the convoy. Players do not directly control the vehicles in the convoy, rather they set paths for the convoy to follow along city streets, while also dropping power-ups such as decoys or smoke-screens to aid the survival of the convoy. Players control a convoy of vehicles investigating anomalies around sections of a downed alien spacecraft, which are protected by various types of defensive towers that have to be destroyed. The style of the gameplay has been described as "reverse tower defense", "tower attack", and "tower offense". Convoy has blue health bars while tower is red Extensive experiments on both a toy dataset under controlled settings and real datasets demonstrate that our method significantly outperforms existing methods, which validates the effectiveness of our sRNN-AE method for anomaly detection. To facilitate anomaly detection evaluation, we also build a large-scale anomaly detection dataset which is even larger than the summation of all existing datasets for anomaly detection in terms of both the volume of data and the diversity of scenes. We further improve sRNN-AE in the following aspects: i) rather than using a predefined similarity measurement between two frames, we propose to learn a data-dependent similarity measurement between neighboring frames in sRNN-AE to make it more suitable for anomaly detection ii) to reduce computational costs in the inference stage, we reduce the depth of the sRNN in sRNN-AE and, consequently, our framework achieves real-time anomaly detection iii) to improve computational efficiency, we conduct temporal pooling over the appearance features of several consecutive frames for summarizing information temporally, then we feed appearance features and temporally summarized features into a separate sRNN-AE for more robust anomaly detection. Further, to reduce the computational cost in alternatively updating the dictionary and sparse coefficients in TSC optimization and to alleviate hyperparameters selection in TSC, we stack one more layer on top of the TSC-inspired sRNN to reconstruct the inputs, and arrive at an sRNN-AE. The optimization of sparse coefficients in TSC with the Sequential Iterative Soft-Thresholding Algorithm (SIATA) is equivalent to a special stacked Recurrent Neural Networks (sRNN) architecture. Specifically, in light of the success of sparse coding based anomaly detection, we propose a Temporally-coherent Sparse Coding (TSC), where a temporally-coherent term is used to preserve the similarity between two similar frames.
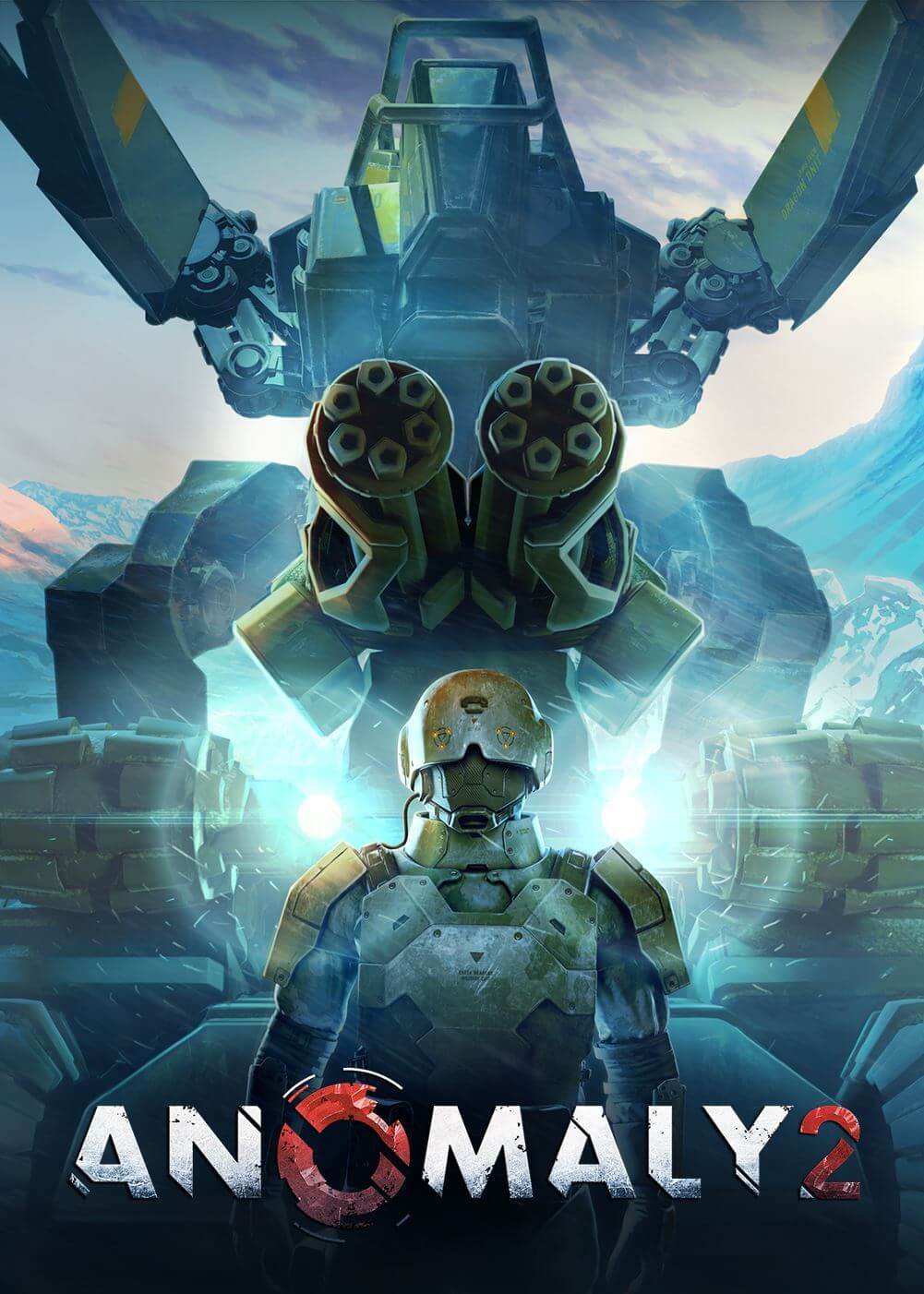
This paper presents an anomaly detection method that is based on a sparse coding inspired Deep Neural Networks (DNN).
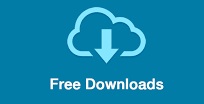